This is the Sponsored paywall logged out
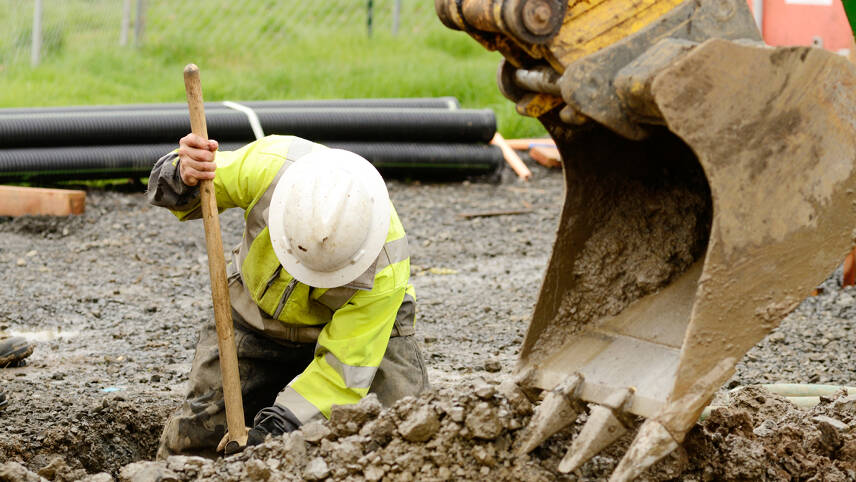
Predictive maintenance promises better asset management as well as lower cost of ownership, more uptime and availability, and preventing problems with machinery before they become critical. But how prepared are utilities to implement it, and what are the fundamentals they need to have in place before they do? Liam Knapp, chief commercial officer at Peacock Engineering, a part of global asset management company BPD Zenith, has the answers.
There are fewer companies doing predictive maintenance than companies talking about it.
There’s no denying that when it comes to asset management, predictive maintenance is the Holy Grail. However, if you are a big field-based business with legacy assets that are tens of years old – or more – you are unlikely to have the equipment in place or the data capability and availability to execute predictive maintenance.
On the other hand, there are some organisations that have the latest assets, for example equipment from Siemens or from Vanderlande featuring data models for organisations to reach predictive maintenance, but there is work still to be done to join that data up with asset hierarchies.
For instance, you might have a turbine covered in sensors, enabling a data scientist to use data from the turbine to predict failure, but the process needs to be supported by the ability to act. Warning that a failure is imminent is one thing, but good asset management processes are required to prevent failure.
Robust operational processes are essential for organisations to be able to respond effectively, and even more so if they are field-based. Another challenge lies in if your assets are widely distributed, for example in a power generation network, and of varying ages, types, and conditions. The organisation needs to understand the complexities of the logistics of getting people and spare parts to the asset, including the types of vehicles needed and also the lead times to complete the fix. In short, the response is much more complex.
Even with good condition monitoring and failure prediction established, along with the right work management processes and technology to respond, organisations must still consider asset lifecycle and investment. Is it better to repair, or is the asset at its end of life? Is there any need to replace the fundamentals of asset management?
What’s the story with my data?
Many companies are still building their asset management and work management processes. A good maintenance system relies on effective mobile working, spares, and scheduling. That’s along with the traditional asset management focuses of compliance, lifecycle costing, and replacement at end of life.
One of the issues you hear from customers – not just in utilities – is that people know they want to get from asset management to predictive maintenance, and most know what data they need. However the challenge lies in the feasibility, and finding a commercially viable way of getting that data.
What’s important to them? Is it vibration analysis? Is it oil analysis? At what frequencies do measurements need to be taken? How should we structure the data against our asset hierarchy? Unfortunately, there’s no blueprint to follow; every asset base is different.
The criticality of assets varies depending on the organisation, including the operational impact on output and services should the asset fail, where the asset is located and the difficulty in getting to the location, and if the asset is nearing the end of its lifecycle.
Key to understanding your asset base is determining the individual and collective asset importance and what data you need to collect. For example, we have a client in the waste-to-energy sector that has very successfully implemented condition monitoring – the step before predictive maintenance.
The customer burns gas from landfill to create power and has a lot of remote sites. Getting engineers out to those locations on time is tricky and expensive, so they decided to monitor equipment remotely and collect lots of SCADA data at a central point.
That information can be fed into an AI scheduling system which tracks where technicians are in the field using vehicle telematics. The system will then select the optimal person to go and do the job.
In the case of that customer, the business was already collecting the correct data for asset monitoring, but a lot of companies will have data in siloes and won’t have joined it up in a structured model.
Indeed, feedback we often hear from CIOs is that they have all this data and can’t unlock it. We have used our depth and breadth of experience to create products to accelerate that journey. Once you have the data, you can consider developing a genuine digital twin, which, in the true sense of the word, isn’t a virtual representation of your facilities, but a digital, real-time representation of the health of your assets.
Enterprise asset management: Getting the basics right
At Peacock Engineering, part of BPD Zenith, a leader and specialist in delivering enterprise asset management (EAM) solutions, we know that no two asset estates are the same and the needs of businesses vary. Understanding your asset base, depreciation, and cost of maintaining those assets at a very granular level is where asset management can empower better decision-making, routing people efficiently, understanding your inventory and spares, generating work orders, servicing assets efficiently, and being more productive.
Compliance is essential, whether it’s regulatory, or health and safety, or your ability to adhere to the standards of your insurance. An asset management system should fulfil all these functions.
The next step in EAM is monitoring, where you might have integrations with external systems such as a SCADA system. This allows you to study aggregated sensor data and create a health score for the asset. When you understand asset health, you can then act upon that information and drive improved business outcomes.
Predictive maintenance is only useful if it can drive improved outcomes. Organisations must create the ability to intervene and positively affect asset performance.
Predictive maintenance: Aiming high, starting small
The asset management space is evolving. It’s gone from maintenance, which was viewed as an overhead, to seizing the opportunity to be more competitive. It’s become a differentiator for businesses rather than a fulfilling part. With the maturing of the asset management sector, there is opportunity to provide new services to customers.
Our experience is that the number of customers who are anywhere near realising the full benefits of predictive maintenance is relatively small. For those that are, a good foundation in work management and understanding inventory availability is crucial. If you are aiming for predictive maintenance, start with a documented maintenance strategy that drives the business case for predictive and defines the data you need and the processes you want to automate.
It is important to ensure you have leadership and shared vision for the project in the organisation. Get those fundamentals right, and you’ll avoid failure, helping to blaze a trail as predictive maintenance becomes a reality in utilities.
For more information on Peacock Engineering’s enterprise asset management solutions visit peacockengineering.com or contact the team on 020 8038 7511.
Liam Knapp is the chief commercial officer at Peacock Engineering Ltd. Having joined the company in 2012, Knapp has an extensive knowledge of managed service, mobility, fingertip mobile, HSQE, scheduling, and system validation, and works closely with clients in the energy and utilities, facilities management, pharmaceutical, and logistics industries.
Peacock Engineering is part of the global asset management solutions company BPD Zenith, a specialist in delivering innovative technologies that help large and medium-scale organisations better deliver against their asset management strategies. An IBM Platinum Business Partner, Peacock Engineering works with organisations in the utilities and energy, oil and gas, facilities management, transport and logistics, life sciences, manufacturing, and defence sectors.
Please login or Register to leave a comment.